STANDARDIZATION NEWS
Cleaner Cannabis: Standard Targets Contamination
One of the challenges in the emerging cannabis market is the issue of contamination.
Read More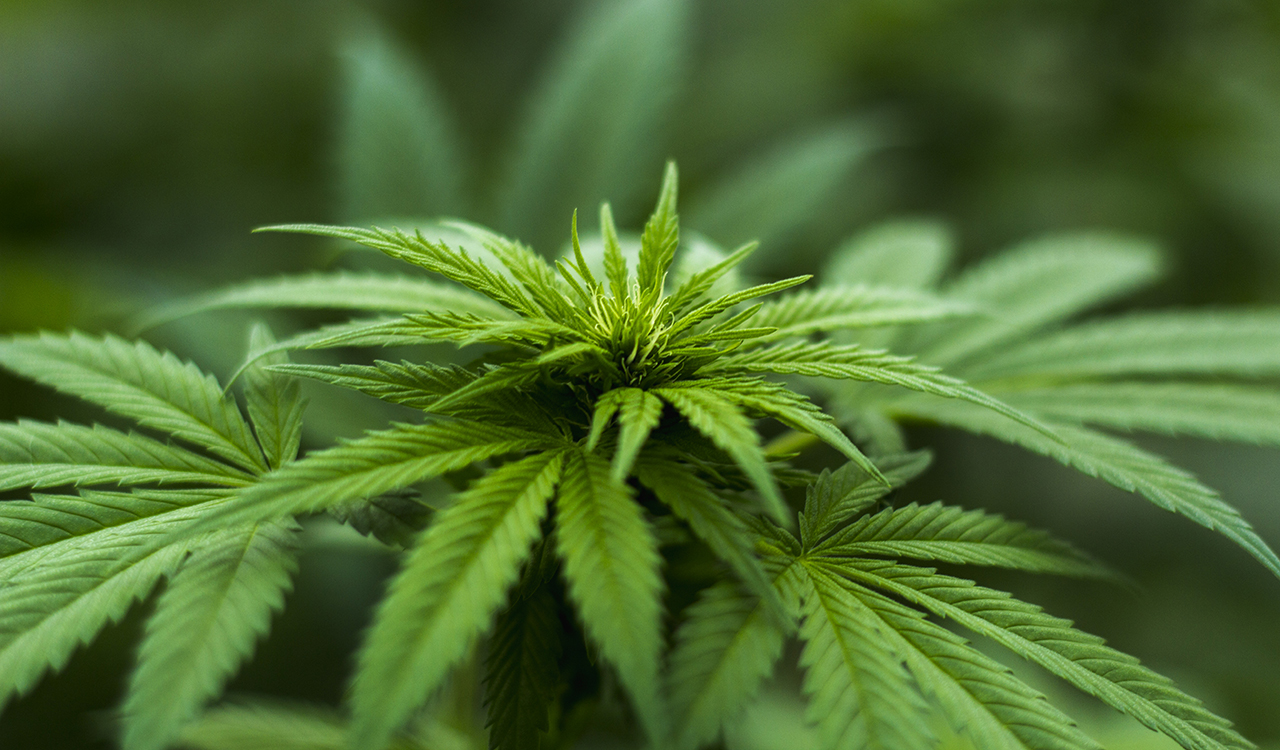
Digging Deep on Coal Standards
Coal has provided a reliable source of power to humanity for thousands of years. Standards have helped make it part of today’s mix of energy sources.
Read More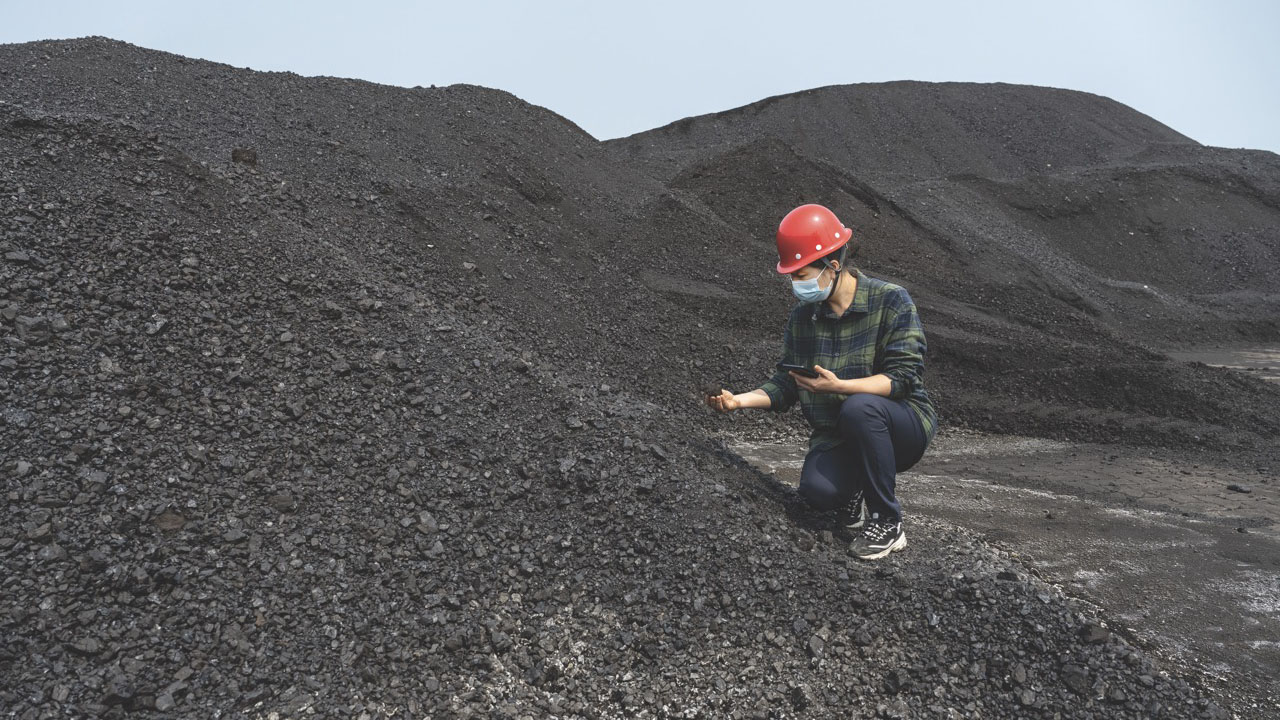
STANDARDIZATION NEWS
July / August 2025
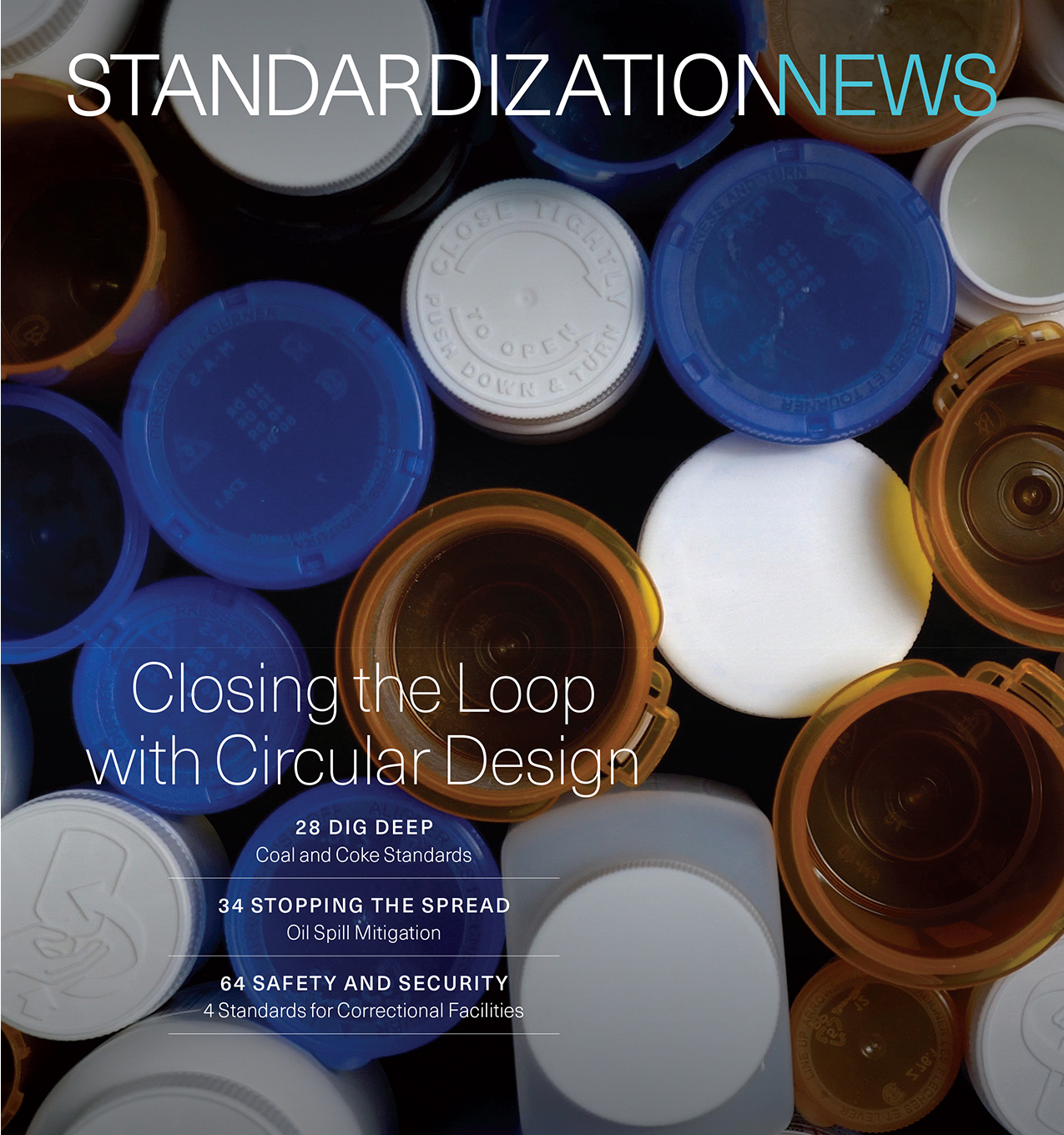
Featured Stories
Latest Articles
Looking for the ASTM International News main page? Click here.